인공지능(AI)
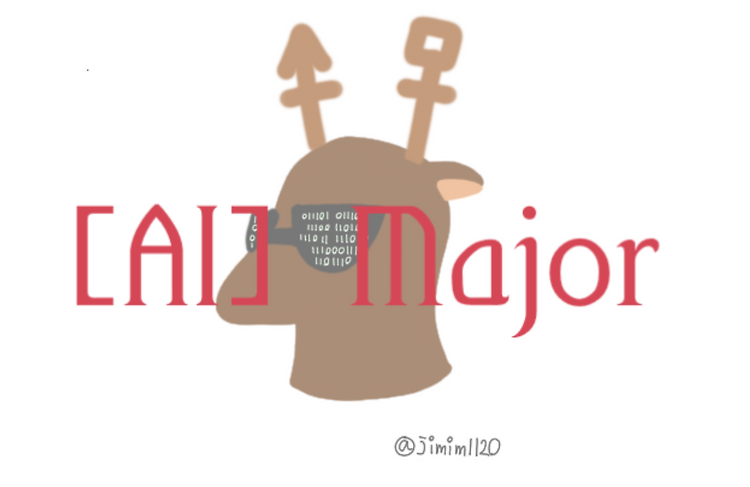
03 Analytic Geometry
(해당 강의노트는 Marc Peter Deisenroth, A. Aldo Faisal and Cheng Soon Ong, 『Mathematics for Machine Learning』을 기반으로 작성하였습니다) 03 Analytic Geometry: 해석 기하학 목차 Norm & inner product Orthogonality Orthogonal projections Rotations 1. Norm & inner product 1.1 Norm - Definition: 벡터 공간 위의 norm은 실수로 가는 mapping function임 1. Manhattan norm - 모든 벡터의 manhattaon norm이 1인 end point를 가진 그래프 - 파란 벡터의 manhattan norm은? : ..
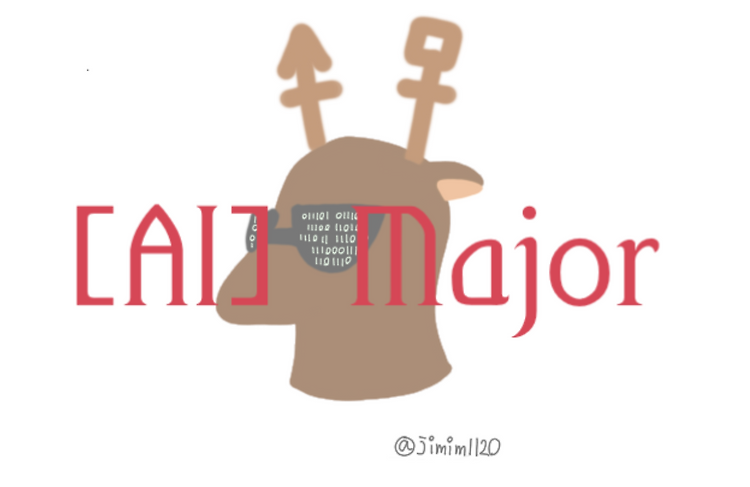
02 Linear Algebra
02 Linear Algebra: 선형대수학 목차 Introduction Vectors, vector spaces & matrices Linear independence & rank Linear equations 0. Introduction 0.1 Motivating Example Finding the relation of a house size with its price The size of house size i $$a_i$$ The price of house $$b_i$$ Find $$x_1 :slope, x_2 : intercept$$ 여기서 붉은 선은 모델, X는 데이터를 나타냄 아래의 두 미지수를 찾는 것이 목적 (모델을 만들기 위해), 모델의 파라미터에 해당 $$x_1, x_2$$ 선형모델은..
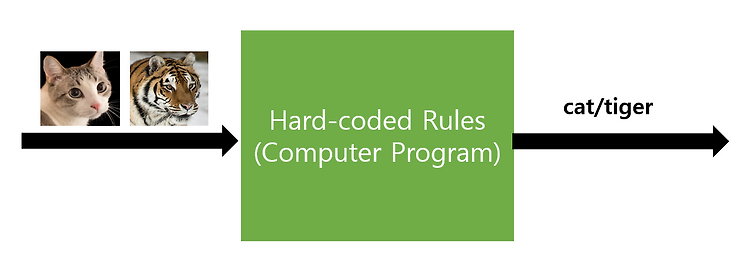
01 Introduction AI Basic: 인공지능, 기계학습이란?
01 Introduction AI Basic: 인공지능, 기계학습이란? 기계학습(Machine Learning, ML) 명시적인 규칙 없이 기계가 데이터로부터 학습하도록 하는 기술로, hard-coded(fixed) 규칙을 사용해 프로그램을 짜는 전통적인 컴퓨터 프로그래밍과는 구분되는 개념. 기계학습의 세 가지 키워드 기계학습 기계학습을 위한 수학 Data 데이터 Image, text, languages, time series Vectors, matrices, tensors Model 모델 - Linear models - Deep neural networks - Kernel machines - Probabilistic models - Linear algebra - Probability Training a..
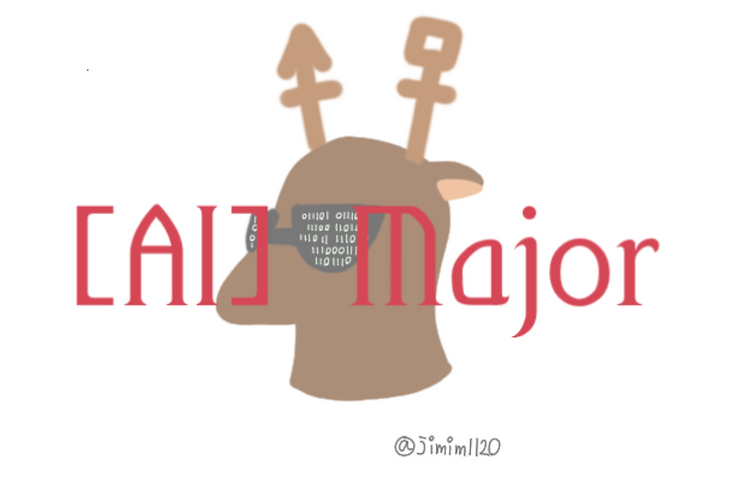
00 인공지능 발전사
00 인공지능 발전사 목차 컴퓨터란? 인공지능이란? 인공지능의 역사 인공지능의 현재 1. 컴퓨터란? 컴퓨터는 끊임없이 발전하고 있지만 그 종류는 오직 하나이다. 1936, Turing Machine : 실제로 구현되진 않았지만 컴퓨터를 최조로 수학적인 모델로 표현 1948, von Neumann architecture : 현재까지도 컴퓨터의 구조로 채택되고 있음 2. 인공지능이란? AI : Artificial Intelligence By Wikipedia Intelligence demonstrated by machines 기계로 구현된 지능 By Investopedia The simulation of human intelligence in machines that are programmed to thin..
![[논문리뷰] 적대적 생성 신경망 : Generative Adversarial Nets(GAN)](https://img1.daumcdn.net/thumb/R750x0/?scode=mtistory2&fname=https%3A%2F%2Fblog.kakaocdn.net%2Fdn%2FPORZX%2FbtqI2G4Vd23%2Fn7o8svLi6rAzN9wjn1Uadk%2Fimg.png)
[논문리뷰] 적대적 생성 신경망 : Generative Adversarial Nets(GAN)
논문 리뷰 Ian J.Goodfellow, [Generative Adversarial Nets] 목차 Abstract Introduction Related work Adversarial nets Theoretical Results Experiments Advantages and disadvantages Conclusions and future work 논문리뷰에 앞서 알아둬야 할 함수, 변수, 기호 D : 판별 모델 output : 0~1 하나의 값으로 스칼라에 해당G : 생성 모델 output: 확률p : 확률 분포 p(z) : z 입력 시, 해당 변수의 분포를 나타냄θ : paremeter(weight) θg : 생성 모델 가중치 θd : 판별 모델 가중치z : random seeds 0. Abstr..